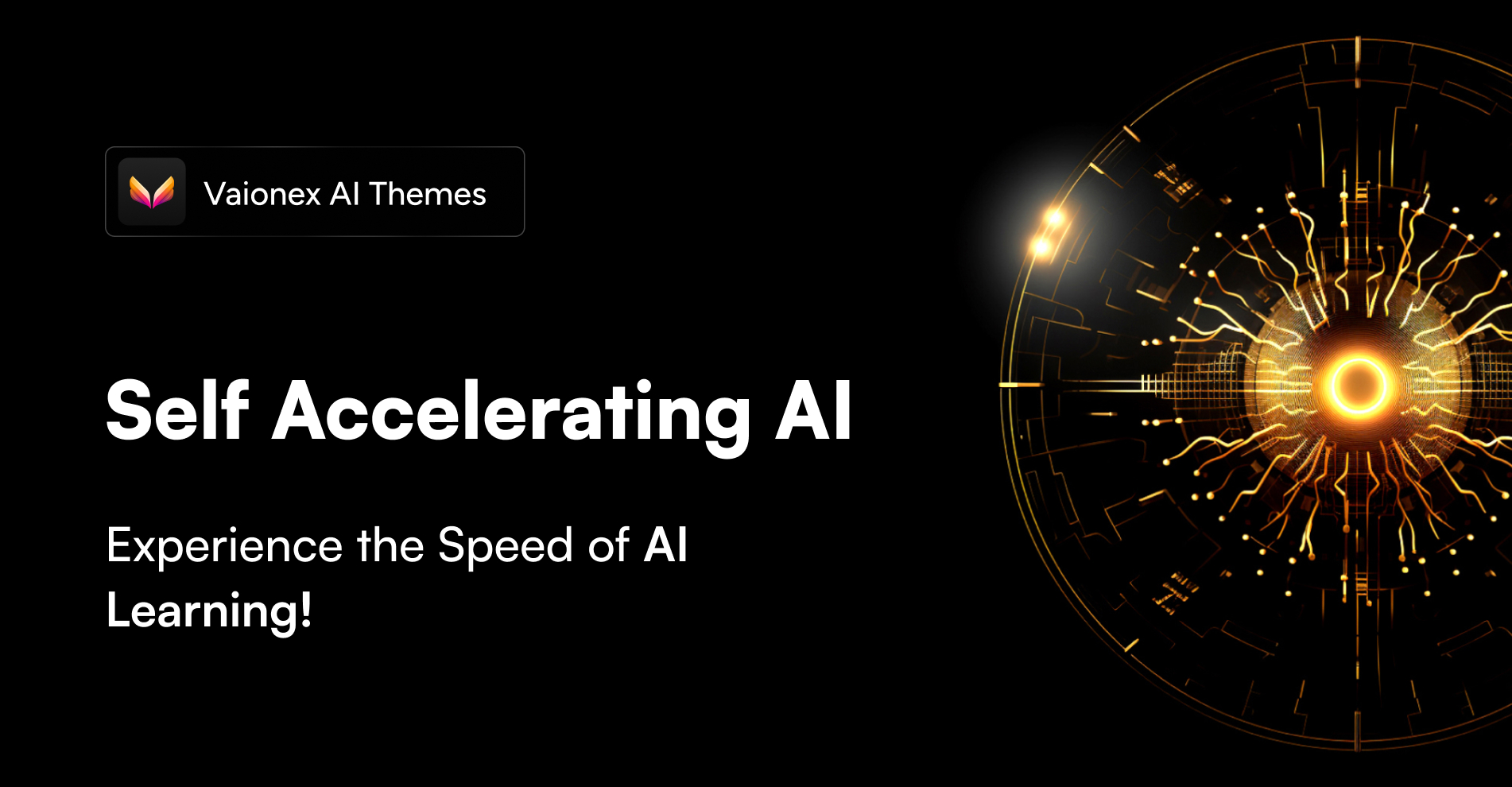
In the ever-evolving world of artificial intelligence (AI), one of the most exciting developments is the advent of self-accelerating AI. This AI structure holds the promise of not just improving, but exponentially enhancing the user experience through the principles of caching and optimisation.
Rooted in the idea of learning from repeated interactions, self-accelerating AI improves response times each time it interacts with the same low-level model (LLM). The underlying goal? Delivering fast, efficient, and increasingly refined responses.
Self-accelerating AI is a form of artificial intelligence that uses a feedback loop to improve its own performance, accuracy, and efficiency. This is achieved by learning from repeated interactions and then leveraging caching to store and retrieve data more quickly.
The self-accelerating AI is designed to “remember” the results of past computations and interactions, thereby reducing the need for time-consuming recalculations. The design of self-accelerating AI also involves optimisation strategies, which streamline processes and tasks, making the system faster and more efficient.
Optimisation allows the AI to evaluate various possible solutions and select the most efficient one, thus improving performance over time.
Self-Accelerating AI and LLM
Low-Level Models (LLMs) represent the more basic models within a hierarchical AI system. They generally deal with simpler tasks and computations, which act as building blocks for more complex processes.
In the context of self-accelerating AI, these LLMs play a crucial role. With repeated interactions, the AI system can develop a better understanding of the process and outcomes, effectively learning the most efficient way to operate. This learning is then stored in a cache for future use, allowing the AI to recall and apply these methods when the same or similar task arises, thus accelerating its response time.
Caching and Optimisation
Caching and optimisation are essential to the effectiveness of self-accelerating AI. Caching allows the AI to quickly recall information from past interactions. This results in a significant reduction in computation time as the AI can skip the step of recalculating the same information.
Optimisation, on the other hand, enables the AI to streamline processes by identifying the most efficient course of action based on past experience. This process of learning and improving allows the AI system to provide increasingly refined responses, thus enhancing the user experience.
Running GPT-4 at an enterprise level can be a costly endeavour, particularly for extensive tasks that require substantial computing resources. With an impressive ability to process 10 million requests per day, the costs can escalate to a few million dollars per year.
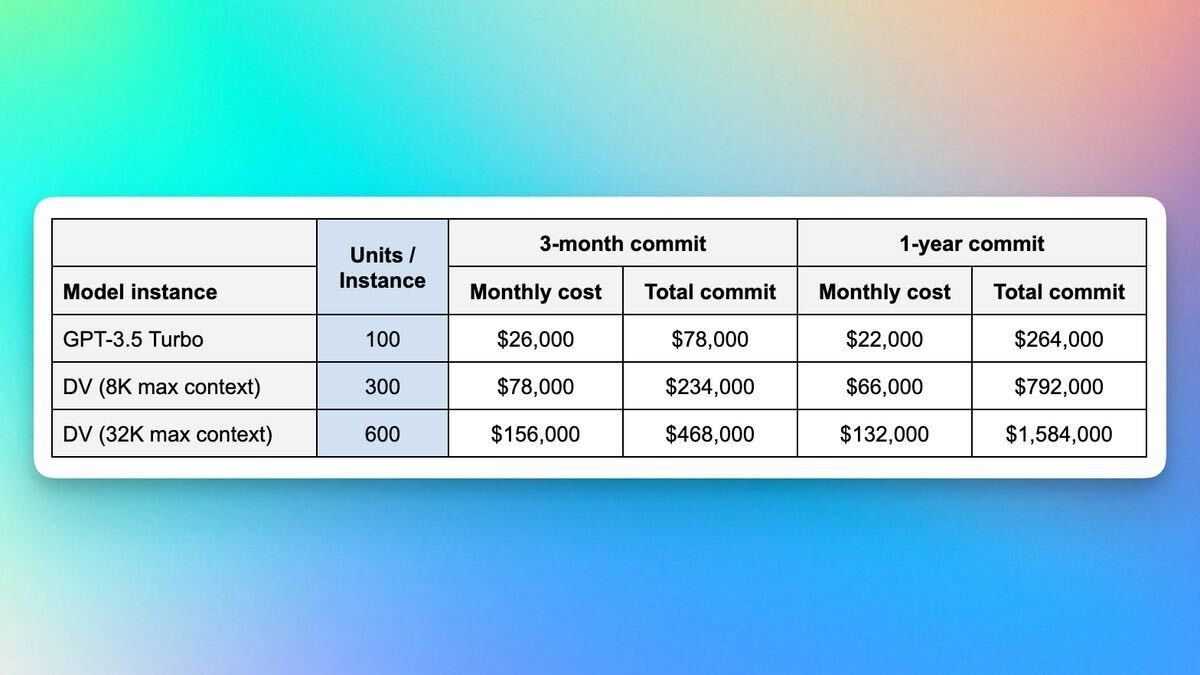
This price tag encompasses the computational power, infrastructure, and maintenance needed to support such large-scale operations. While the real-time capabilities and raw processing power of GPT-4 provide unparalleled benefits in various applications, the financial aspect can be a limiting factor for many businesses.
In contrast, using a cached version of GPT-4 for handling the same volume of requests – 10 million per day – can significantly reduce costs to mere hundreds of dollars. By employing cached responses and utilising pre-computed information, the need for real-time processing is diminished, thus cutting down on computational requirements.
This approach may lose some of the dynamic responsiveness of a full-fledged GPT-4 implementation but offers a financially viable solution for enterprises that require the analytical capabilities of the model without the hefty price tag.
The cost-saving transition from millions to hundreds of dollars without sacrificing the core functionality demonstrates the flexibility and adaptability of GPT-4 in meeting various business needs and budgets.
Impact and Applications
The potential applications of self-accelerating AI are vast. For example, in customer service chatbots, self-accelerating AI could be used to quickly retrieve previous interactions, allowing the bot to provide personalised responses at a faster pace. In computational settings, it could significantly speed up calculations by caching results and optimising solutions based on prior computations.
Moreover, self-accelerating AI could be particularly useful in fields such as healthcare, where AI is used to analyse patient data and suggest treatment plans. In these settings, the ability of self-accelerating AI to recall past interactions and provide faster, more efficient responses could save valuable time and potentially improve patient outcomes.
The Future of Self-Accelerating AI
As AI continues to evolve, self-accelerating AI presents an exciting path towards improved performance and efficiency. The combination of caching and optimisation strategies creates a system that learns, adapts, and improves, providing an ever-enhancing user experience.
It’s clear that the future of AI lies in its ability to self-accelerate, offering unlimited potential for advancement across multiple fields and industries.
Vaionex AI Engine
As an innovating force in the AI space, Vaionex has incorporated self-accelerating AI into its robust infrastructure. Using this advanced AI model, we have made significant improvements in the efficiency and speed of deploying new AI tools & apps.
The self-accelerating AI allows Vaionex to create more intelligent, responsive, and user-friendly applications by learning and adapting from user interactions and system requirements.
These self-learning capabilities have enabled us to deliver faster response times and continuously refine and improve the user experience. Vaionex is creating innovative solutions that are redefining the boundaries of what apps can do and shaping the future of technology.
Get in touch with us today at one@vaionex.com to learn more about our AI capabilities.